In a previous article, we discussed how real-world evidence (RWE) can facilitate and accelerate major breakthroughs in healthcare and life sciences. We also alluded to the challenge that lies in unlocking the value of RWD/RWE while preserving data privacy and complying with security regulations that control the use and analysis of useful and oftentimes, mission-critical, datasets. Process-driven workarounds like data de-identification combined with technologies such as tokenization work to a point but still need to be faster and more extensive. By partnering with Duality Technologies, Globant has taken the lead in supporting clients with an RWE solution that streamlines the use and maximizes the value of RWD, no matter who has it or where it resides. Through such innovative partnerships, Globant lives up to its mission to reinvent the healthcare and life sciences industry through tangible technology-driven solutions.
Challenges – RWD/RWE and Data Privacy Regulations
The big hurdle for the success of any RWE study is data. RWE studies need large volumes of diverse RWD for analysis, but such access and collaboration is hindered and even blocked by data privacy regulations and security and governance concerns. In addition, utilizing disparate datasets to their full potential means having full utility—linking disparate data sets, schema matching, etc.—and high efficiency to increase the speed at which insights are generated. When it comes to the traditional means of satisfying these requirements, there’s friction that goes against the needs of the teams that rely on RWE for mission-critical insights.
Too Much Time & Effort Before Analyses Begin
This is important for two reasons: the first is a risk associated with sharing healthcare data, which is protected by privacy regulations. Entities, such as healthcare providers, who are the custodians of healthcare data, must comply with data privacy and security regulations. To do that, they traditionally de-identify RWD before enabling access to it. However, the effort associated with it, and the risk of re-identification, may influence healthcare providers’ willingness to participate in data sharing, especially if they don’t have the infrastructure, resources, and technological know-how to ensure compliance.
Second is that healthcare data can be vast, complex, and varied. Often, significant data cleaning and harmonization efforts are required after acquisition. If data aggregators don’t handle this task, the responsibility shifts to the sponsor organization’s data management teams. They must prepare the data for analytical tools used by various departments. Instead of concentrating solely on analysis, they spend extra time coordinating these efforts, which affects the return on investment (ROI). Moreover, delays can prolong the time-to-market, losing both competitive edge and significant revenue. Ultimately, it’s patients who pay the highest price for such delays with suffering or even death that could have been prevented.
Diminished Data Quality and Utility
Removing identifiable information from a dataset reduces valuable data context and, therefore, quality, limiting its usefulness for research and insights generation: reducing granularity of data, generalizing unique values, masking outliers, or reducing data linkage potential. When trying to connect data from different sources, for instance, to identify relationships between certain types of cancers and specific genomic markers, the absence of unique identifiers makes this process quite challenging. While tokenization is a useful method for linking de-identified RWD across data sources, it has limitations. Some drawbacks include the high cost of setting up and maintaining a tokenization system, especially one that can handle vast amounts of RWD, and a potential risk of re-identification if tokenization keys are compromised. All this impacts time and effort and still may not pass stricter privacy requirements as found in GDPR.
HIPAA
Health Insurance Portability and Accountability Act (HIPAA) is a U.S. federal law with the primary goals of improving the portability of health insurance coverage, enhancing the privacy and security of patients’ health information, and establishing standards for the electronic exchange of healthcare data. HIPAA privacy rules require that protected health information (PHI) be de-identified before it can be shared for purposes such as research or marketing. Under HIPAA, de-identified data is not considered protected health information (PHI) and is, therefore, not subject to the same privacy regulations as PHI. De-identification is the process of removing personal identifiers such as name, address, and social security number, from the dataset. It is required when the PHI is used for a purpose other than providing healthcare services. To be truly de-identified, data must not be reasonably linkable to an individual and must be verified through expert determination. This process is often a lengthy negotiation among all stakeholders until a balance is found between the data that can be shared versus the usefulness of that data.
GDPR
The European General Data Protection Regulation (GDPR) is a more extensive and stricter than HIPAA. It encompasses a broader range of personal data, emphasizes informed and explicit consent from data subjects for processing their data, and may still apply to de-identified data. In the context of GDPR, data is no longer considered personal data and is therefore not subject to the same GDPR requirements, only if the data has been processed so that it can no longer be linked to an identifiable individual. Therefore, processes more stringent than traditional de-identification – such as pseudonymization or anonymization – may be required to enable healthcare data sharing under GDPR. As one can imagine, the time, cost, and limitations in de-identifying data are far more pronounced with anonymized data.
The Technology Recommended by Regulators
In June 2023, the UK’s Information Commissioner’s Office (ICO) published recommendations, along with case studies (including one that was co-developed with Duality Technologies) showing a clear path to (UK GDPR) compliance and greater efficiency. In their PETs Guide, the ICO shows where and how various PETs satisfy or do not satisfy the major components of GDPR privacy requirements. The guide explains how using PETs can enable insights from datasets without compromising the privacy of the people whose data is in the dataset, and how appropriate PETs can make it possible to give access to datasets that would otherwise be too sensitive to share. Additionally, the UK Information Commissioner has recommended considering PETs, for organizations that share large volumes of data.
Navigating Access to RWD Today and in the Future
Given the rigorous data privacy regulations, such as HIPAA and GDPR, access to RWD and the generation of RWE is a complex issue.
Currently, accessing and using RWD for research often requires obtaining informed consent from patients, ensuring that data is anonymized or de-identified to protect patient privacy, and sometimes undergoing a review by an ethical review board or institutional review board (IRB). These measures are taken to ensure that the data is used responsibly and ethically, and that patient confidentiality is maintained.
Moreover, data governance structures have been put in place to ensure adherence to these privacy laws. These structures outline who can access the data, where, for what purpose, and how the data is protected. The use of secure data environments, also known as data enclaves or trusted research environments (TRE), has become an attractive approach for in-country use of sensitive data. Data enclaves allow researchers to access and analyze data within a secure environment without allowing the data to leave the enclave, thereby maintaining data security and privacy.
As we look to the future, privacy-enhancing technologies (PETs) will further enable and streamline the secure use of RWD. PETs are a segment of technology and specific PETs vary by software and hardware types and the kinds of use cases they can support. In some cases, combining PETs is the way forward. The main types of PETs include fully-homomorphic encryption (FHE), multiparty computation (MPC), federated learning (FL), and trusted execution environment (TEE)s. For more information, here are some examples wherein various health organizations have found the use of PETs useful in accelerating and enabling their work:
- PNAS Paper 2020: Dana Farber Cancer Institute utilizes FHE to link genomic data with oncological datasets.
- DARPA Press Release 2020: DARPA uses FL with FHE for COVID-19 research.
- ASCO paper 2023: Tel Aviv Medical Center utilizes FHE to unlock RWD across borders and accelerate breakthroughs in medical research.
- PNAS Paper 2023: Dana Farber Cancer Institute, Tel Aviv Medical Center, and others combine FHE and MPC for work in Oncological research.
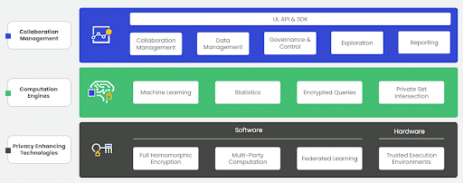
Getting Started
The power of RWD and RWE must be wielded with a deep respect for data privacy and ethical considerations. While technology promises new methodologies to ensure data security and privacy, a comprehensive and shared approach to governance, ethics, and responsible use remains paramount. This balance of innovation and responsibility will ensure that RWD/ RWE continue to illuminate the paths of discovery and healthcare delivery for years to come. As the intersection between R&D and healthcare delivery continues to evolve, it’s clear that RWD and RWE have a critical role to play guiding the way to a more data-informed future in life sciences.
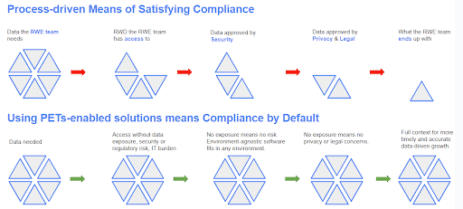
Globant has partnered with Duality Technologies, a company that offers privacy-enhancing technologies, to support our clients in unlocking the value of RWE in a secure and compliant way. We leverage Duality’s privacy-enhancing technologies to develop RWE platforms that enable healthcare data sharing and collaboration across geographies, while respecting local data privacy regulations.
Get in touch to start your journey to secure and compliant data sharing and RWD-driven research.