Deriving value from your AI data strategy
What does data strategy mean for businesses, and what value can we derive from it? Let’s narrow it down to what strategy means in practical terms by leveraging Mintzberg’s 5 Ps of Strategy and how it translates to Data and AI. These examples do not convey their entirety but as a high-level overview.
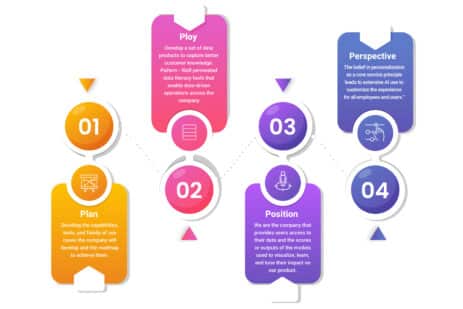
Strategy to me, when you boil it down to first principles, is three things. Number one, it’s an assessment of what you think the truth is today. Number two, it’s a prediction of what you think the truth is tomorrow. And number three, it’s a decision of how you’re going to place your resources amongst any number of different alternatives based on your prediction of truth. Data-Analytics Chief of the 2012 Obama US presidential campaign, Dan Wagner
Through his statement, we see that there is an assessment, a vision, and a decision – these 3 stages define the following aspects, which we will go into detail about:
- Vision
- People’s capabilities and distribution of responsibilities
- Use cases and relevance
- Technology, platforms, and governance
- Timeline
Vision
Let’s start with a vision – what role would data have in your organization? Today, companies strive to be data-led, so what must we do to ensure we rely on evidence to make optimal decisions without being blinded by immense volumes of data? We can’t limit our decisions based on our current data or make a decision first while looking for data to justify our hunch. It is equally risky to say that data is the center of everything we do if we cannot make that a reality.
Turning data into a capital asset is the ultimate goal for every organization – we expect a continuous return on data assets that can be reshaped or reused in different ways to drive value for consumers. We have to ask ourselves if our business model can enable us to produce and capture such distinctive data. Does our value offering create novelty that we can capture in data that leads to capitalization by generating a non-fungible dataset?
There are other aspirations for a vision, such as working to drive efficiencies and product refinement or aiming at breadth over depth. We can also empower decision-makers across the organization to have the tools and access to the data they need and build specialized capabilities from the most demanded areas later. It could even catch up to the digital world and vanquish manual processes for accessing operational-critical information at the front line of our business!
There is no one-size-fits-all as aspiration should harmonize with the other factors we consider- consuming data, product refinement, or empowering decision-makers to have available tools and access to data.
People’s capabilities and distribution of responsibilities
What is data fluency, and what level should you expect from your team? From the basic ability to consume info appropriately to challenging assumptions behind the data and exploiting data to find new insights, it may be difficult to ensure that we have highly data-fluent people who are positively engaged with the business functions where they are placed. Should the organization concentrate the core data capabilities on a team of specialists, which may keep them in silos from what the business needs?
The degree to which we distribute or concentrate expertise, ownership, development, and data usage will impact our capacity to hire and retain people. There are various considerations, such as the organization’s operational efficiencies, practices around governance, and motivations. We aim to define the depth and distribution of data-related expertise in practical terms – can I keep them engaged while encouraging growth within the company?
Use cases and relevance
Although the vision sets the tone, contact points between people and data drive the application of a successful data-driven strategy. While an evolving data strategy finds new use cases, there must be clarity on a set of use cases and products that act as a litmus test on whether the strategy is sound.
It is important to establish the seeds, identify priorities, and create the framework and workflow for data use. From there, we can overview what we need to tackle, who will be responsible, and what information is required. We define data products as digital products with data as their core functionality. We are considering AI models, dashboards, data streams, data-consumption APIs, and more!
Use cases are about enabling the effective use and adoption of data for users in a manner that will make them feel compelled to leverage capabilities based on their merits. So how should we build this list? We can focus on decisions by looking at Marr’s framing of possible objectives in these three main aspects, better business decisions, improved operations, and data as an asset. There are nuances in how we consider improving processes as a case of applying decisions, such as designing products customers want best or even choosing the best logistics route. Data is an asset that enables businesses to offer actual value when we define appropriate data products that genuinely leverage data. By focusing on who and how business decisions are made and the information required, we can pull from a single thread to align the rest of the objectives.
We would want to consider these factors:
- Technical viability
- Business viability
- Usability
- Cost to try/ Build
- Klout/ Political cost to fail
- Clarity of success criteria
- Overall vision alignment
- Required conditions and the likelihood of achievement
- Business/Adoption gain from success
We can prioritize the remaining cases by eliminating options based on meeting the required conditions. The most relevant thing, to begin with, is the ‘dependency relations’ between these initiatives, as ordering these factors will affect the overall likelihood of success and adoption.
Technology, platforms, and governance
Data strategy requires a digital substrate, a set of processes to govern its operations, and decisions on building blocks of technologies, languages, and services. A data platform is a central component that addresses many of these concerns. It is the cornerstone of a good data strategy that enables data products to operate.
Large-scale decisions such as leveraging open-source technologies or services like PaaS/SaaS enable us to scale quicker but hinder differentiating factors or proprietary ecosystems. We can also consider typologies of data platforms, data lakes, warehouses, data lakehouse, data fabric, and mesh. These sets of decisions may impact the technological capabilities available to the business. The type of data processes affects storage like SQL, NoSQL, HDFS, individual binary files, etc.
Identifying the right data platform to manage resources is particularly important for MLOps. We should decouple this decision to have a layer of control for budgeting – a thoughtfully designed data platform considers the interplay of use cases, people, visions, and technologies.
Timeline
Roadmaps are essential to provide the team with a framework for success to help define the desired outcome. The question revolves around the dependencies between elements and our capacities to develop them. We can apply agile methodologies where we deliver value in small increments. The main goal is to provide value as quickly as possible with iterations based on feedback and adoption. As we find that some assumptions do not hold, we would need to adjust the course.
Building the timeline needs to consider these checkpoints and define, based on our current knowledge, whether the milestone has provided value. During each stage, we need to evaluate different value tracks. Are we trying to transform the whole organization to provide a network effect on how to work leveraging data? Are we focusing on a minor test case to drive adoption by example? Are we onboarding new team members with different skill sets needed for the developments? We will consider breadth vs. depth for a healthy roadmap with checkpoints.
Companies with a smaller risk appetite may not move fast enough and lose momentum or support with their stakeholders as the incentive for transformation is not enough.
A fluid plan is hard to actualize, but paying attention to your roadmap, helps set conditions for that spark.
Closing the loop and ongoing efforts
One contentious aspect of the strategy is no matter how many times we interpret it, it is treated as a one-off effort whose outcome is no more than a heavy slide deck without continued execution. A proper functional strategy needs to be a constant ongoing transformation track that can be intense at times, requiring monitoring and assessing.
Some aspects need to have a higher frequency, particularly at high seniority levels within the organization. The data strategy revisions could cover the following:
- What has changed in technology in terms of evolution and deprecations
- What has changed in needs and use cases
- What has changed in business strategy, and how does it impact the data strategy
- How well are we managing our current roadmap, and what are the pain points
- Getting enough buy-in from stakeholders and evangelizing across the organization
- Critical business questions list – which will become the next batch of use cases
- Future-scaping the organization to have a longer-term input to the vision
- How to establish effective feedback loops within the organization, data usage, and the aspects of the strategy
A good data strategy is a live endeavor – a continuous transformation program. It may have a starting point with much more focus and intensity, but the only way to maintain a strategic advantage is to consider it as the backbone of the way we operate in the data world.