Late cancer diagnosis and treatment had plagued our society for years. Many have lost their battle to various forms of cancer. Either the detection was inadequate or the treatments procedure were inefficient. But with Machine Learning, we are looking at paradigm-shifting diagnosis and treatment options of cancer. Not only that, it can accurately determine the possible outcomes of the treatment. Artificial intelligence is key to early diagnosis
Cancer is a disease of the genome, caused by certain mutations of the DNA. It results in different gene expressions of the person. Machine Learning models can be trained and used to detect specific mutations and predict the likelihood of cancer development for the patient. But what’s the technology behind this?
Neural Network as an early stage detection tool
Image recognition and analysis is one of the first applications of Machine Learning. If we look into the healthcare data repository, most of the diagnosis contains digital imaging. Convolutional Neural Network (CNN), a specific Machine Learning architecture, is well equipped to analyze images. It evaluates pixel-level data of images, accounting, and recognizing dots, lines, and curves. The analysis is so precise that it can detect and classify objects within images. Furthermore, the CNN mechanism can be trained to predict outcomes of applying chemotherapy and immunotherapy on a variety of malignancies.
Companies are trying to build a low invasive screening test for cancer detection by drawing blood samples and using ML to analyze them.
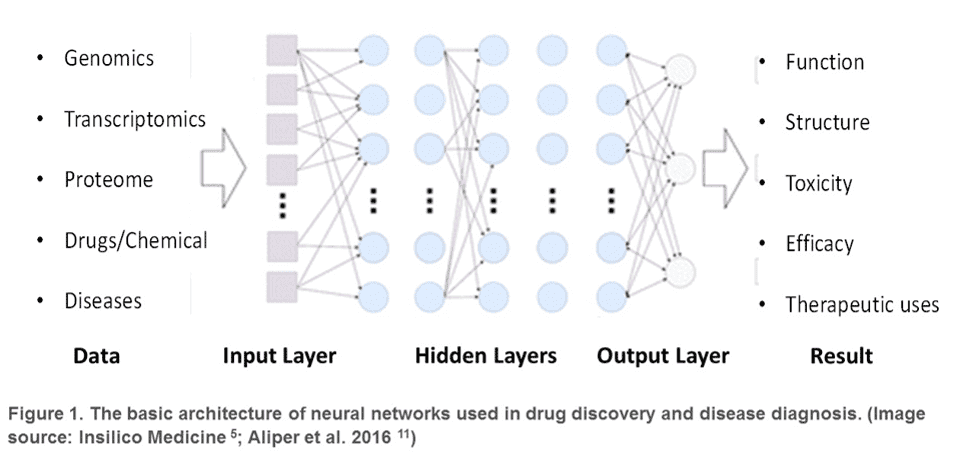
A Simple Representation Of Very Complex Architecture for Drug Discovery and Disease Diagnosis
Neural networks for treatment procedure
Another domain that is ripe for ML algorithms application is a drug development and clinical trial. It takes enormous time and money investment into developing drugs and performing clinical trials. AI can synthesize patient data and clinical trial data to get an appropriate test case. Most importantly, AI can test a drug’s efficiency before clinical trials.
NNs can be trained on genomic and chemical properties to predict cancer cell sensitivity to therapeutics. Hence it can be used to find out relevant investigational drugs for a particular patient’s cancer management. These algorithms can recommend personalized treatment that is more likely to yield positive outcomes, using DNA blueprint or RNA response signatures.
NNs can come handy to interpret genetic variations and patterns to decode cellular processes. A procedure named CRISPR is currently being experimented on to see if gene mutation can be reverted. Not only can AI read and analyze large sets of medical data much faster than a human. It can also more accurately determine the results of a patient’s treatment options and possible outcomes of the treatment.
Simple Workflow of an intricate and complex neural network architecture
The advancement in AI paves the way for the personalized medicine development platform.
Developing effective personalized medicine can be fast and pocket-friendly for both manufacturers and patients. Machine Learning can calculate and process drug combinations and evaluate it with patient’s biological signatures. This process is done on a virtual simulation environment. Its a cheaper, quicker and efficient process to verify the efficacy of the drug on the disease. In a very short time, ML can suggest a drug formula that has a higher probability of clinical trial success.
Machine Learning can guide doctors to undertake targeted treatments for patients. A data repository of patient’s DNA characters and cellular history and various sources of medical data like clinical trials and tests makes it possible.
Using Deep Learning – a form of Machine Learning, few US clinics have started delivering personalized radiation therapy to patients. Deep Learning algorithms can access and analyze digital images, coupled with the patient’s clinical risk factors. The algorithm produces a different radiation dose for each patient. This method has proved to reduce the adverse effects and failure rates of treating cancers.
Simple schematic representation of personalized radiation technical workflow
The beauty of the methodology of these models is that they are adaptable and adjustable according to different clinical data and is a closed loop process.
Since most of the NN framework is data-driven and adaptable, each healthcare providing facility can modify the system using their data sets.
There is no doubt that Artificial Intelligence tools can individualize multiple cancer treatment options and is a leap forward in personalized medicine. However, to make the tool successful, it needs reliable data from all the nodes of the healthcare ecosystem. Like FDA, drugs and pharmaceutical companies, biotechs, academia, AI companies, diagnosis companies, clinical trial companies, and others. Hence a collaboration among all of these distributed components is required to drive innovation forward.
Source
–https://ai-med.io/machine-learning-treatment-cancer/
–https://towardsdatascience.com/machine-learning-is-the-future-of-cancer-prediction-e4d28e7e6dfa
–https://www.cancernetwork.com/oncology-journal/artificial-intelligence-oncology-current-applications-and-future-directions/page/0/1
–cancer.gov/about-cancer/treatment/research/car-t-cells
–https://www.forbes.com/sites/nicolemartin1/2019/10/18/how-artifical-intelligence-is-advancing-precision-medicine/#3a3bc958a4d5
–https://healthitanalytics.com/news/ai-brings-personalized-cancer-treatment-to-cleveland-clinic