It’s a busy conference season already. This month we’ve been to CES, and we also got back recently from the International Plant and Animal Genomics Conference. The PAG conference is one of the world’s premiere events on the subject. We had the chance to attend the whole week of conferences! It was a great opportunity to support our partners who were presenting their work, and connect with other colleagues. We got a glimpse of all that’s state-of-the-art in academia/the industry, as well as looking into what is coming, where the genomics business is going, and the most relevant innovation cases around the globe.
Before we dive in, let me lay some groundwork. The insights from this conference have a lot to do with breeding, and so here’s a little intro into the relationship between breeding and genomics research.
First of all, what is breeding and how is it related to genomics?
Breeding is the activity of studying and applying ways of enhancing certain organisms (plant or animal) to achieve some desired characteristics, called “traits.” Traits could be, for example, a resistance to certain fungus or climate changes, retaining more nutrients, being taller, etc. We talk in terms of parent and child in breeding, but we’re obviously not referring to humans.
There are two general types of breeding:
- Conventional: This is when you cross two organisms (called “hybridization,” when talking about plants). It’s the primary technique for creating variability to identify and select the most desirable organism in regards to certain traits of importance.
- Unconventional: This type of breeding can be divided into two subtypes:
- Non-genetically modified: Including DNA information (molecular markers) as part of the process of evaluating and selecting the child’s DNA with the desired characteristics.
- Genetically modified (GM): Involves artificially engineering the DNA, cutting off or adding specific genes (usually from bacteria), that will produce the desired characteristics (e.g. pesticide resistance) of the child’s organisms.
Conventional breeding purely deals with making decisions based on observable characteristics of the organism. For example: how tall they are, how resistant they are to fungus, etc. It usually takes more time, since the breeder has to wait for the plant to develop and grow in order to make the measurements.Using molecular markers for the decision making (MAS: Marker-Assisted Selection) involves analyzing the organism at a DNA level in order to make better and faster decisions. This requires more sophistication. This is where genomics and the study of genes play a huge role.
Data and AI as the stand-out trends at PAG
In two particular ways, technology took center stage at the event this year. I noticed first a big emphasis on data collection and analysis, but also saw genomics beginning to look to AI for some assistance in important research. Here’s what I noticed at PAG.
Data can take research further
The trend in genomics this year seems to be high-throughput & high-volume data collecting and analysis. Researchers are also focused on creating new algorithms, techniques, devices, and technology capable of capturing as much data as possible for further processing. The areas of main focus are:
- Genotyping: Scanning multiple areas of the DNA and combining results together, in order to infer relationships between the identified genes from the organism and the trait of the living organism. They use cutting-edge techniques to analyze massive data patterns in order to look for not-yet-known relationships in the DNA. These relationships, for example, can explain why a plant is resistant to certain microbes.
- Phenotyping: Taking massive measurements from the organism (plants for example) that can be used later on to correlate lab results with desired characteristics expressed on the organisms. This serves to prove a working theory as true or false. For this particular category, innovation comes mostly from new devices such as drones or robot data collection devices.
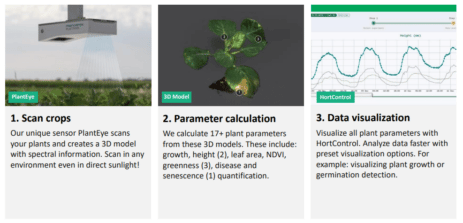
Of course, collecting more data (especially in the form of high-res pictures) requires ever-better data management. It now has to support hundreds of terabytes of information per day. This is giving rise to a new line of research, seeking for a way to deal with this volume of data efficiently. It’s research that is gaining traction as a result of having more and better high-throughput techniques.
Data sharing and cross-collaboration
One thing that dominated conversation at the PAG Conference is that there were many workshops and talks on facilitating collaboration between different research labs. The motive behind this push is to achieve something bigger than what each one could achieve individually. I heard the term “federated” in several talks, referring to group collaboration and data sharing between different hubs. Another indicator that caught our attention is the names and sponsors of each paper that groups presented. It’s common this year to see 5-7 authors and co-authors on papers, and more than one lab involved in the publication, which is aligned with this federation initiative.
Software updates at PAG
Other indicators we observed on these topics at PAG are related to software artifacts:
- 3 years ago people set a contract (API) as standard, in order to interchange breeding information from different systems. This makes collaboration and data sharing somewhat easier and more efficient. They created the BrAPI (Breeding API) standard, and now more and more companies and research labs are starting to integrate into their work and cite in their papers.
- To make experiments more robust and less error-prone, we see a constant effort to improve the MIAPPE (Minimum Information About Plant Phenotyping Experiment). With a general consensus of the minimum information needed to perform a relevant and robust experiment for the scientific community, the time needed to biocurate and fill the gaps will be reduced, which, at the end of the day, means easier sharing and experiment reproducibility.
- There are a number of public databases (RefEx, TreeGen, CartograTree, Germinate, RiceBase, Chado, etc) that are free to use and are constantly improving. The main purpose is to centralize bio-curated, high-quality information in a standard format, with the hopes of research work and proving solutions to gene annotation, data visualization, data export and more.
- We attended some workshops where were they introduced new (and existing) solutions in order to facilitate the adoption of automated tools. The reason for using the tools is mainly for data pipelining and processing that will save the scientist time, and therefore money from their funding. What those new tools have in common isn’t just the purpose they want to achieve, but also the extendable functionality they offer. They are flexible enough to adapt to other open source tools and specific lab needs. Here are a few examples:
- BMS (Breeding Management System): one of the most used platform to run breeding programs
- PhytoOracle: data pipelining
- Galaxy project: platform for all type of bioinformatics tasks
- JBrowse: genome browsing
- PGAP: genome analysis pipeline
- RefEx: gene analysis
AI deep learning for a faster and automated phenotyping
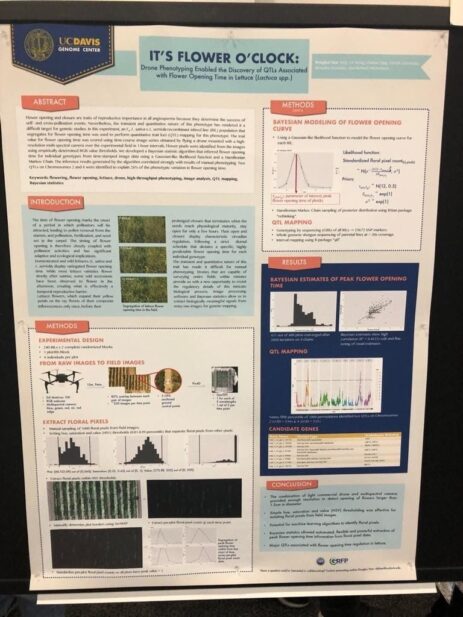
As we saw at PAG, there seems to be an increasing interest from the community in using deep learning and image processing. Even though I saw many uses of this technology (we will see them in a second), the basic idea is to create a pipeline where some device (could be a drone) will collect information from the field (pictures among others) and feed them to a trained neural network, that in the case of images, will process them in order to infer information from them (leaf width, plant length, length from ground to the first leaf, flowering time, etc.), that can be stored for later use by some other model, usually a statistic one.
One proven use of deep learning is to uncover intrinsic relationships between data attributes. That is, provided a set of attributes (called features in the AI world) and a result, the model can infer what relationships between the features can lead to the observed result. This will be especially useful in the phenotype prediction area, where the goal is to determine how the combinations of the genotype and environment may produce an observable characteristic on the organism. For example, discerning which combination of genes and environment will make the plant more resistant to strong winds.
Someday we will see papers on that topic, but for now, deep learning is being used on more reduced-scope tasks, like the following examples:
Fruit classification
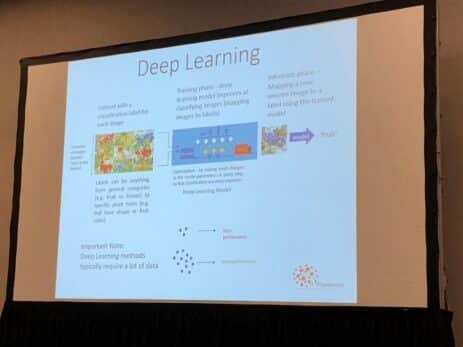
This deep learning model is used to detect when fruits are ready for harvesting. It uses an image from an input, taken from a robot field data collector. These types of problems are usually addressed using a convolutional neural network architecture.
ImageBreed end of season prediction
ImageBreed is a solution to predict end-of-season for Maize using deep learning applied to ortho-mosaic images taken from a drone. The pipelines rely on an open-source solution called BreedBase. Here they use it to store all the information collected from the drone.
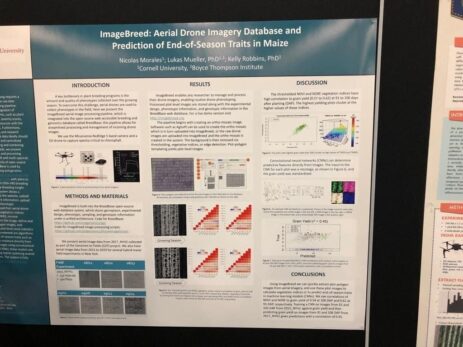
It is then cross-referenced with other information (genotype, experimental design, etc). As a result, they found a high correlation between indices that measure vegetation health (NDVI & NDRE) and grain yield, which took place 91 to 106 days after planting (DAP). The breeder can use this to get an early heads-up of the upcoming task (preparing for the harvesting for example).
Phenotyping based on leaf image processing
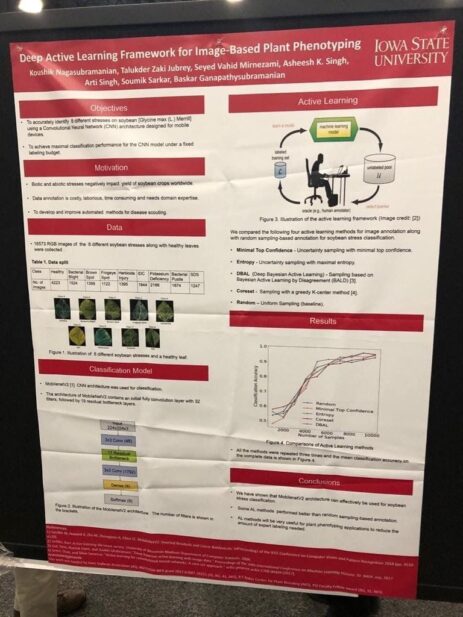
Another deep learning solution (this time from academia, Iowa State) to phenotype plants faster, and automatically. Here, they trained the model with different stressed and healthy soybean leaves, to learn what they look like. This allows it to automatically label new leaves with a high level of confidence. No additional expert needed. As you can see on the bottom-right, some of the classification methods achieve >95% precision.
PAG Conclusions
PAG was a great place to be this year. The advances and new collaborations never cease to impress. The open source community is getting bigger and stronger, being the “de facto” standard for new and modern software. Whatever research these bright minds are up to, there is an important emphasis on collaboration and federation across the board. People are developing new technology in order to capture more and more data, moving towards data-driven decisions. And AI will gain more traction in this direction in the immediate future.
Thankfully, The Bill & Melinda Gates Foundation provides huge funding for most research on global hunger, climate change, and genome discoveries. That is one of the big reasons to keep tabs on this research. These advances in technology and genomics might very well become the answers we need to the questions climate change brings. My interest in this conference has only grown through the past years. It’s increasingly important to be kind and keep the planet in mind as we research and develop technologies. If you have the chance to go to PAG next year, give it a try. The combination of technology and discovery will surprise you!